Artificial Intelligence to improve in-stock levels
I recently attended Groceryshop 2023 and unsurprisingly, the agenda and the exhibit hall were filled with discussions and solutions positioning Artificial Intelligence (AI) as a panacea to drive operational gains throughout the grocery retail value chain from CPG supply chain to grocery checkout.
Artificial Intelligence as a means to pinpoint stock-outs was a significant part of the conversation, which is an obvious signal to me that many are in the market evaluating this use case for AI, one that is validated by the business cases for AI stock-out detection I am building with leading grocery retailers.
These business cases include obvious variables such as required CapEx and OpEx and labor efficiencies gained by faster shelf data capture.
One less obvious variable is data accuracy.
I am a recovering grocery operator who spent 30 years at major grocery chains leveraging technology to solve the complex operational challenges that bedevil our industry.
So, let’s just say I’ve learned that an ounce of asking the right questions of a technology provider up front can be worth a pound of post-implementation fixes.
To help you ask the right up front questions related to data accuracy, here’s a primer on AI stock-out accuracy based on my recent experiences with leading retailers and CPG brands.
Different flavors of AI shelf-data capture deliver different levels of stock-out accuracy
What you see in Figure 1 is an accuracy comparison between different shelf inventory data capture technologies and processes. The results of this comparison are from a tier 1 grocery retailer who evaluated the “practical accuracy” of different “gap scanning” approaches based on their own manual trailing audit of 20k items across multiple stores.
Computer vision is the type of AI that enables a computer (in this context, a device with a camera in a grocery aisle) to identify and understand objects in images and videos (while also automating manual tasks done by “error prone” humans, in this case assessing shelf-inventory conditions).
Data accuracy improvements driven by computer vision compared to legacy manual solutions are intuitive.
Less intuitive is the difference in data accuracy between motion-based computer vision and still image-based computer vision.
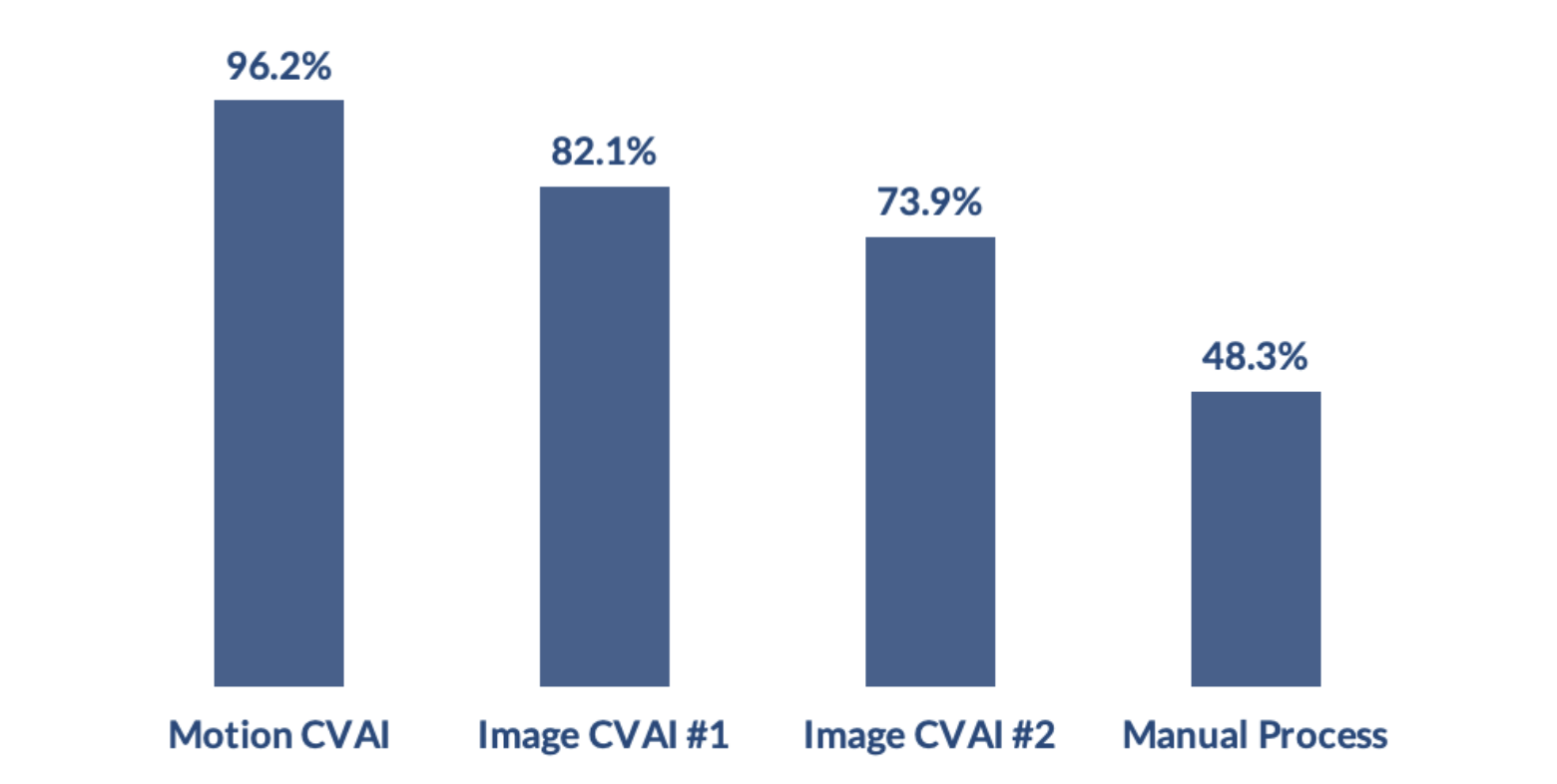
Figure 1
Motion-based computer vision data capture provides dozens of images of each item on the shelf, from multiple angles and perspectives, seeing the shelf much as a human does.
These numerous views provide the Artificial Intelligence analyzing the item with the ability to correct for partially blocked or turned products and human error.
The accuracy of motion-based computer vision stands in stark contrast to the much lower accuracy level delivered by image-based computer vision data capture that takes a single snapshot of items on the shelf where seeing all items from one angle is nearly impossible.
When evaluating AI-based shelf data accuracy claims, read the fine print
Mark Twain is attributed with saying “Facts are stubborn things, but statistics are pliable”.
If you’re evaluating AI-based shelf intelligence solutions, you’ve likely heard statistics from solution providers touting 90%+ accuracy levels.
Here’s a breakdown of how different solution providers define accuracy to get to those statistics:
First off, the way to measure real shelf intelligence data accuracy is by manually auditing system results in a real store environment. This accuracy is “practical accuracy”.
As mentioned above, a leading retailer used this approach as part of their evaluation process to gather the facts needed to validate shelf data accuracy statistics claimed by shelf-intelligence solution providers.
From a shelf intelligence solution provider perspective, some providers measure stock-out “system accuracy” defined as how accurately a shelf intelligence solution identifies if a product is on the shelf and available for sale through the eyes of a customer.
Many providers measure “theoretic accuracy” defined as how accurately their shelf-intelligence solution recognizes a product on the shelf based upon a system image library.
In other words, without ever looking at the actual shelf, a solutions provider will look at known items in their system where the picture quality was high enough to be processed. Then they measure how many times they were right.
While both definitions may produce claims of 95%+ data accuracy, the first delivers insights that will have a much larger impact on achieving your ultimate business objectives of increased sales and customer satisfaction.
Building your AI-powered shelf intelligence business case
Whether you’re a CPG brand or a grocery retailer, AI-powered solutions to help you more effectively and more efficiently understand your shelf inventory conditions have reached a level of maturity that warrants your consideration.
Leading CPG brands and retailers alike are currently deploying shelf-intelligence solutions to give themselves a strategic advantage in an increasingly competitive market.
As you evaluate shelf intelligence solution providers and gather the facts for your business case, I hope this perspective on shelf data accuracy from my experiences with the AI-powered shelf-intelligence early-adopters that went before you will be valuable.
This article is by Mark Abernathy, Head of Retail at Pensa Systems. As a 30-year grocery retail veteran, Mark brings a wealth of expertise helping CPG brands and retailers accelerate growth and improve customer satisfaction through innovation and disruption management.